chapter 5 Getting a sense of Big Data and well-being
Big data – a new way to understand well-being?
“Big Data”, was cited 40,000 times in 2017 in Google Scholar, about as often as “happiness”!
(Bellet and Frijters 2019)
The datafication of social life has led to a profound transformation in how society is ordered, decisions are made, and citizens are governed.
(Hintz and Brand n.d., 2)
Digital devices and data are becoming an ever more pervasive and part of social, commercial, governmental and academic practices.
(Ruppert et al. 2013, 2)
The majority of Big Data are collected in a different way to the national surveys and interviews we encountered in Chaps. 3 and 4, and consequently has numerous different qualities. One is that surveys and questionnaires are, by and large, overt methods, in that it is obvious you are asking questions to generate data. The new technologies use data which are collected covertly and so often gathered on individuals without their ‘considered consent’, and are often processed without transparency. Figure 5.1 shows just a small selection of the types of personal data that are useful and valuable for social analytics and that are covered in this chapter.
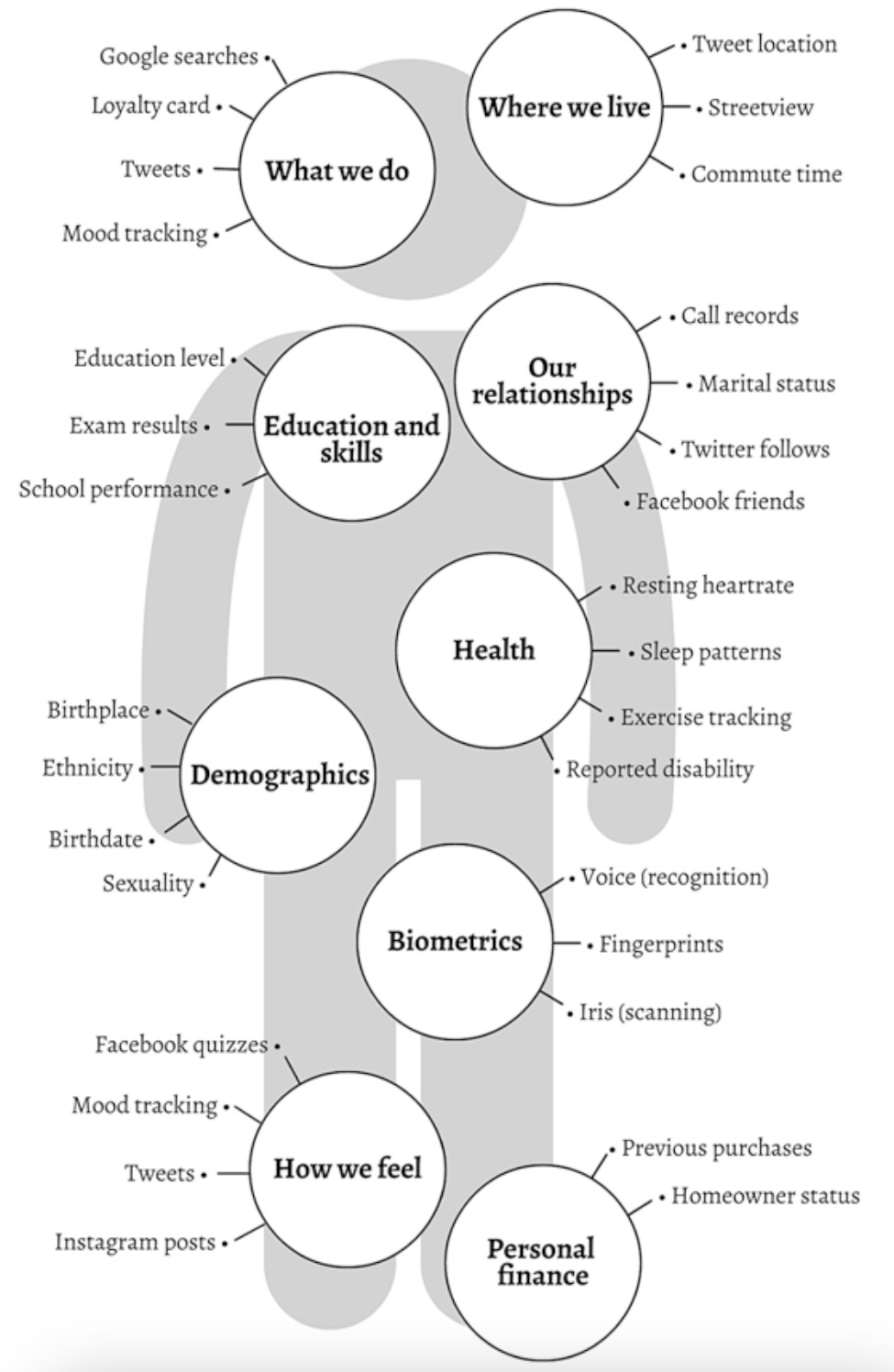
Fig. 5.1 Some examples of personal data used for social analytics in the era of Big Data
Social analytics involve the: monitoring, analysing, measuring and interpreting of data about people’s movements, characteristics, interactions, relationships, feelings, ideas and other content. Figure 5.1 shows only a few of many more examples. Here, they are categorised into domains that share the same names as the UK’s well-being measures, to enable you to cross reference the different kinds of insights available under each domain from these data (although biometrics is a new addition). The data are from ‘observations’ of how we move around the on and offline world. They can include behaviours collected by sensors (think of how your mobile phone uses data via GPS to tell you when the next bus is, or that you are about to encounter traffic on the motorway). They include our feelings, shared by social media data, or in apps. While demographic data have long been collected, as we know, these newer forms of data can say much more about us, our well-being and quality of life. As we shall discover, this is both for good and bad and any insights gained need to be put into context.
As we have also discovered, data are not only numbers or text, but can be sound and pictures. Analysing these kinds of qualitative data as Big Data holds new possibilities. In some ways it is these new possibilities that feel the most uncomfortably non-human. Whether it is concern that your phone is always listening to you, or, rather, that Alexa or Siri are (to humanise these technologies). Even the Street View option of Google Maps allows us to look at other people’s homes. I remember keenly finding the image of the flat I rented in London for years, only to see my washing-up through the kitchen window. I couldn’t help but think, I wish I had known they were coming.
More notable than my neglected washing-up being on public view for judgement are other visual data used for training datasets, particularly for facial recognition. There are the moments when you know that facial recognition technology is being used: to log in to your phone, or at passport control at the airport, perhaps. However, they are also being developed for schools, public transport systems, workplaces and healthcare facilities1. Revelations about its use in shopping centres prompted media and public outrage, regulatory investigation and political criticism2. These reactions are in part about the further encroachment on the way we live (like the call centre example from the 1990s that opens the book) and in part the lack of consent and knowledge about these data being collected about us.
Some people who uploaded photos to Flickr, some 10–15 years ago, more recently discovered they (as in the people’s faces and their photos) appeared in a huge facial-recognition database called MegaFace3. They found the database held facial data on around 700,000 individuals, including their children, and was being downloaded by various companies to train face-identification algorithms. These algorithms were then being used to track protesters, surveil terrorists, spot problem gamblers and spy on the public at large3. Notably, a colleague who read this chapter before publication—a digital sociologist,((A digital sociologist is interested in understanding the use of digital media (often data) as part of everyday life, and how these various technologies contribute to patterns of human behaviour, identity, relationships and social change.)) no less—confessed to me their shock at reading this anecdote, as they had used Flickr and were not aware of this story. Therefore, not only are our personal data collected and used without our knowledge, but the controversies surrounding their re-use are not even shared with users. This poses questions for accountability and transparency.
The questions of who is collecting these data, and who is using them, and for what, present a more complex issue than before. Public support for the police to use facial recognition technology is conditional upon limitations and subject to appropriate safeguards, but there is no trust in private company use4. As we have been discovering—it is the contexts of data collection and uses that we need to understand: it is the who, what, where, why and what for? that are important.
- Ada Lovelace Institute 2019 [↩]
- Denham 2019; BBC 2019 [↩]
- Hill and Krolik 2019 [↩] [↩]
- Ada Lovelace Institute 2019 [↩]